Data orchestration simplifies the management and flow of your datasets, empowering you to use your data to its true potential. Understanding how to utilize data orchestration tools and processes effectively can transform chaos into clarity. Discover strategies, tools, and best practices to help your organization make faster, better decisions with data orchestration — let's dive in!
A data orchestration approach involves the strategic organization and integration of various data sources, allowing for centralized and streamlined data management. This includes everything from gathering raw data to cleaning and sorting it to ultimately utilizing it to make informed decisions. With the amount of data being generated today, effective data orchestration is crucial for organizations aiming to achieve a competitive advantage. The global data orchestration tool market is expected to reach $1.3 billion this year and grow to $4.3 billion by 2034, growing at a compound annual growth rate (CAGR) of 12.1%.
Definition and Purpose of Data Orchestration
Data orchestration is a crucial concept in data management and analytics. In simple terms, it refers to the process of centralizing and automating data pipelines for efficient data management. This can involve tasks such as moving data from siloed environments to a centralized repository, managing and administering different data pipelines, and automating various processes related to these pipelines.
Let's break down each aspect of data orchestration in more detail.
Centralizes administration of data pipelines: Organizations today deal with huge amounts of structured and unstructured data generated from various sources such as databases, sensors, social media platforms, etc. This leads to the creation of multiple silos within an organization where different teams or departments have their own separate sets of tools and processes for managing their respective datasets. A data orchestration tool brings all these individual pipelines under one roof, making it easier for organizations to manage them from a centralized platform.
Such centralization improves the overall efficiency of handling data pipelines and reduces the risk associated with scattered datasets. With all the pipelines in one place, monitoring their performance and identifying any issues that may arise becomes easier.
Automates Data Pipeline Processes: Orchestration tools automate various processes for managing multiple datasets, including loading raw or processed data into storage systems, processing large volumes of streaming or batch data at scale, transforming it into usable formats for analysis, and scheduling regular backups.
These tools eliminate manual intervention in these tasks by utilizing predefined rules and workflows set up by users. This saves time and minimizes human errors that could occur during manual operations.
Moves Siloed Data to a Centralized Repository: Most organizations have siloed datasets across various departments due to their different storage systems. Moving them all into a centralized repository through data orchestration tools helps with data consistency and standardization and allows for easier access to merged datasets for analysis. A Gartner study found that 87% of businesses have low business intelligence (BI) and analytics maturity due to scattered and siloed data.
Also Read: What Is Business Intelligence?
Core Steps in Data Orchestration
The process of data orchestration involves a series of core steps essential to efficiently managing large volumes of data. This section will discuss the three main steps in data orchestration.
1. Organizing Data from Different Sources
The first step in data orchestration is gathering relevant data from internal and external sources. With the increasing volume, velocity, and variety of data generated nowadays, it has become crucial to have a centralized system for managing all the information. This includes structured and unstructured data such as customer feedback, product reviews, sales reports, social media interactions, etc.
A robust data management tool can help businesses easily collect and organize this diverse information into a single repository or database. The goal is to have all the necessary information readily available in one place to be accessed quickly whenever needed.
2. Transforming Data for Uniformity and Analysis
Once the data has been gathered from various sources and placed in a central location, it needs to be transformed into a standardized format suitable for analysis. It is necessary to transform these raw datasets to align with each other according to predefined rules set by businesses using tools like ETL (extract-transform-load) or ELT (extract-load-transform) approaches depending upon requirements. These processes enable businesses to convert their raw input into more accessible formats that analysts can easily understand while ensuring consistency across all collected datasets.
3. Activating Data for Immediate Use
After organizing and transforming the data, the final step is activating it. Activating data means making it available for immediate analysis and decision making. Here, data orchestration tools come into play again. These tools have built-in connectors that help businesses integrate the transformed data with various applications, such as business intelligence (BI) tools or analytics software. This integration enables timely access to accurate information, leading to better-informed decisions, improved operational efficiency, and business growth.
Data orchestration plays a significant role in modern-day business operations by streamlining the process of organizing, transforming, and activating vast amounts of data from different sources. With these core steps in place, businesses can acquire their valuable datasets and use them to gain insights that can give them a competitive edge.
Data Orchestration Tools: Key Characteristics and Considerations
Here are some key characteristics and considerations to keep in mind when getting started with your search for a data orchestration solution:
Scalability: One key characteristic of a data orchestration tool is its ability to scale with growing data needs. As businesses collect more data, they need a robust system to manage large volumes without compromising performance. A scalable data orchestration tool should accommodate current and future growth without causing any disruption.
Flexibility: Business needs evolve, and so do the types and formats of data that needs to be orchestrated. The tool should offer the flexibility to connect with various sources, such as cloud-based applications, databases, legacy systems, etc., irrespective of format or location.
Centralized Monitoring: An efficient data orchestration process involves continuously tracking all the moving parts in handling your organization's critical SaaS applications or cloud services from one centralized dashboard, regardless of whether you are scaling up/down or updating/migrating resources within your cloud infrastructure.
Data Security: Effective security measures must be integrated into any successful enterprise-grade solution. Storage and handling of every byte of data being collected and/or repurposed by your team should be in compliance with regulatory standards like ISO, HIPAA, or PCI.
Cost Effectiveness: It's essential to consider the costs involved in setting up and maintaining a data orchestration tool. While some tools offer a one-time license fee, others follow a monthly subscription model. Before deciding, it's crucial to evaluate your budget and the features offered by various tools.
Data orchestration is critical for businesses looking to achieve efficient workflows, make informed decisions, and gain a competitive edge. Organizations should consider these characteristics and considerations when choosing a data orchestration tool.
Common Features of Data Orchestration Tools
Data orchestration tools are essential for any organization that wants to efficiently manage, process, and analyze large amounts of data. These tools offer expansive features that help businesses streamline their data operations and attain insights.
One key feature of a data orchestration tool is its use of Directed Acyclic Graphs (DAGs). DAGs are graphical representations of complex workflows that help visualize the dependencies between various tasks in a workflow. Data orchestration tools use DAGs to create an organized and efficient flow for processing data from multiple sources. This allows users to easily track the progress of each task and identify any potential bottlenecks or errors in the workflow.
Data extraction, transformation, and loading (ETL) functions are essential for enterprises combining data from various sources into a single unified database. Data orchestration tools offer robust support for ETL processes by providing pre-built connectors or APIs that connect to diverse data sources such as databases, applications, files, or IoT devices. These tools also offer advanced task management capabilities that enable users to schedule jobs based on specific triggers or time intervals. This helps automate repetitive tasks and ensures timely delivery of accurate results.
For organizations with established data infrastructure, it is important that their chosen data orchestration tool seamlessly integrates with their current systems without causing any disruption in operations. Most modern orchestrators offer compatibility with various databases like SQL Server, Oracle Database, or Apache Hive, as well as cloud-based platforms like AWS S3 or Azure Blob Storage.
In addition to these common features, data orchestration tools offer advanced capabilities such as data modeling, data quality checks, and error-handling mechanisms. These features ensure the data being processed is accurate, consistent, and reliable. With the help of these tools, businesses can minimize manual errors and focus on drawing valuable insights from their data.
Now, let's check out some of the top data orchestration tools and how they can power up your data game.
Top Data Orchestration Tools
1. Savant
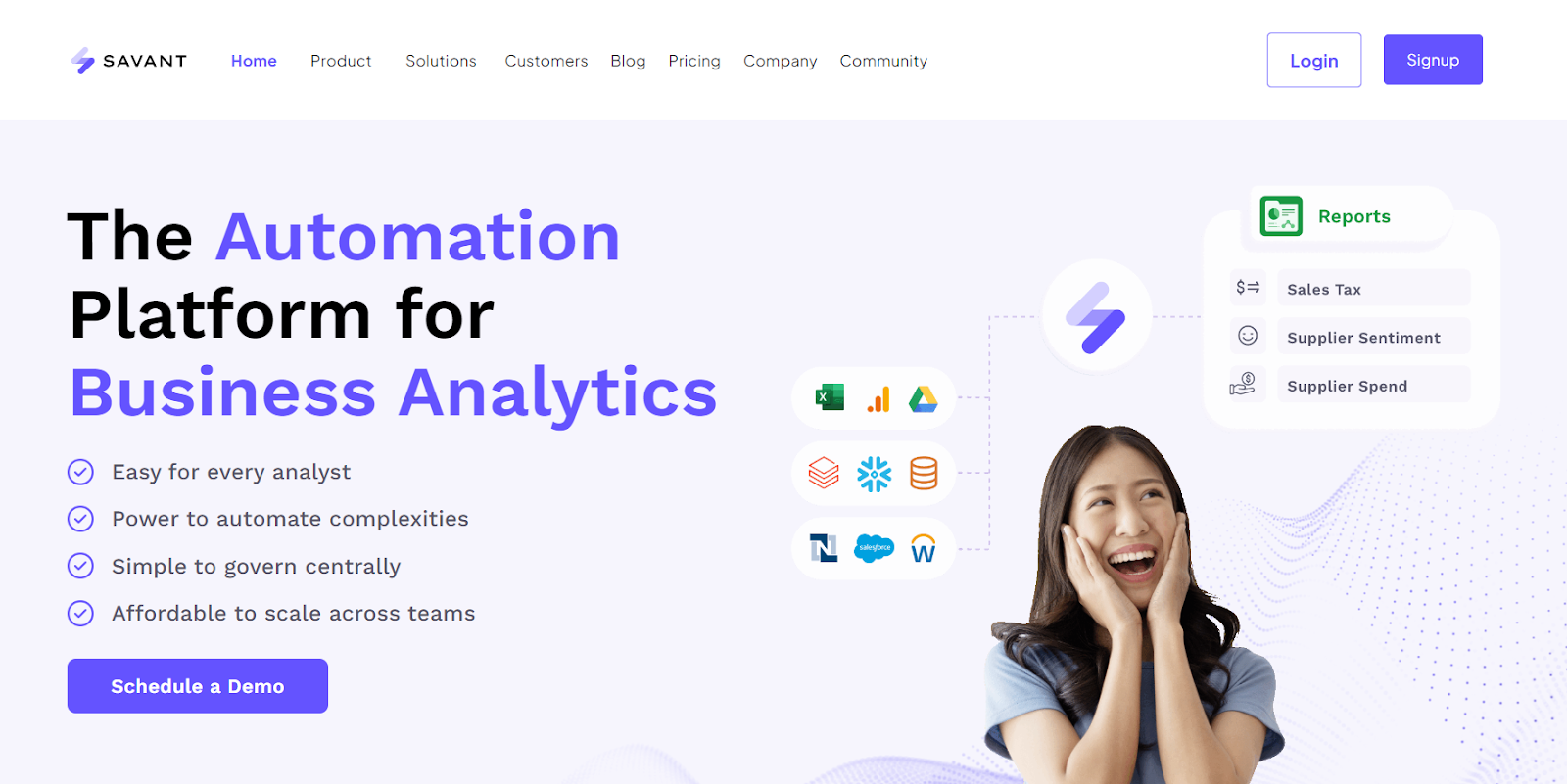
Image Source: Savant Labs
Savant is a cloud-based data analytics and orchestration platform that offers many features like real-time streaming ingestion, automatic schema detection, gen-AI- powered workflow customization, a drag-and-drop interface, and advanced error-handling capabilities. With Savant, users can easily integrate different data sources, including structured databases, unstructured files, or streams from social media platforms like Twitter or Facebook. Its intuitive dashboard provides detailed insights into the status of ongoing jobs and allows for easy monitoring and troubleshooting. Savant implements robust security measures to protect your valuable information from unauthorized access or threats, and its top-notch data cleansing capabilities ensure the quality and accuracy of your datasets.
Arrive Logistics has experienced impressive growth with the help of Savant's no-code data flow automation. It has opened up significant revenue opportunities through data-driven insights. In one month, the company identified over $500K in new revenue through self-service analytics, eliminating around 400 hours of manual analytical work each month and allowing data teams to prioritize more strategic initiatives. This also resulted in a 25% decrease in data requests to engineers and is expected to bring similar savings in data synchronization and replication expenses, highlighting the positive impact of streamlined data orchestration on operational efficiency.
Take the first step towards seamless integration and elevate your data management strategy. Book a free demo today!
2. Apache Airflow
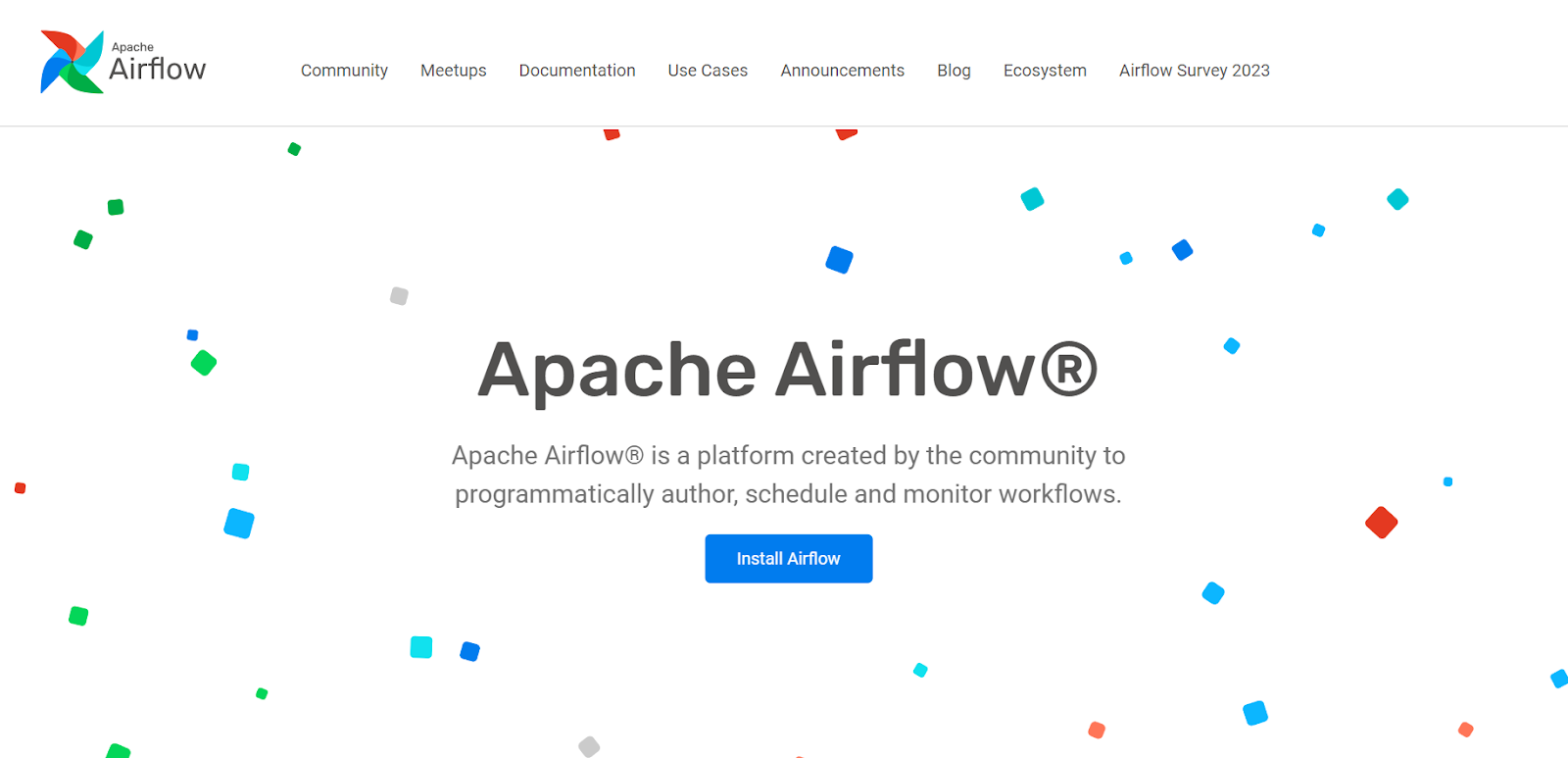
Image Source: Apache Airflow
Developed by Airbnb in 2014 as an internal project before being open-sourced in 2016, Apache Airflow has become one of the most popular workflow management tools in recent years. It allows users to create complex data pipelines using DAGs. These DAGs define dependencies between tasks, allowing for parallel execution while providing features like task retries and scheduling. Airflow supports different execution engines, including Kubernetes, to scale seamlessly with your growing needs.
3. Prefect
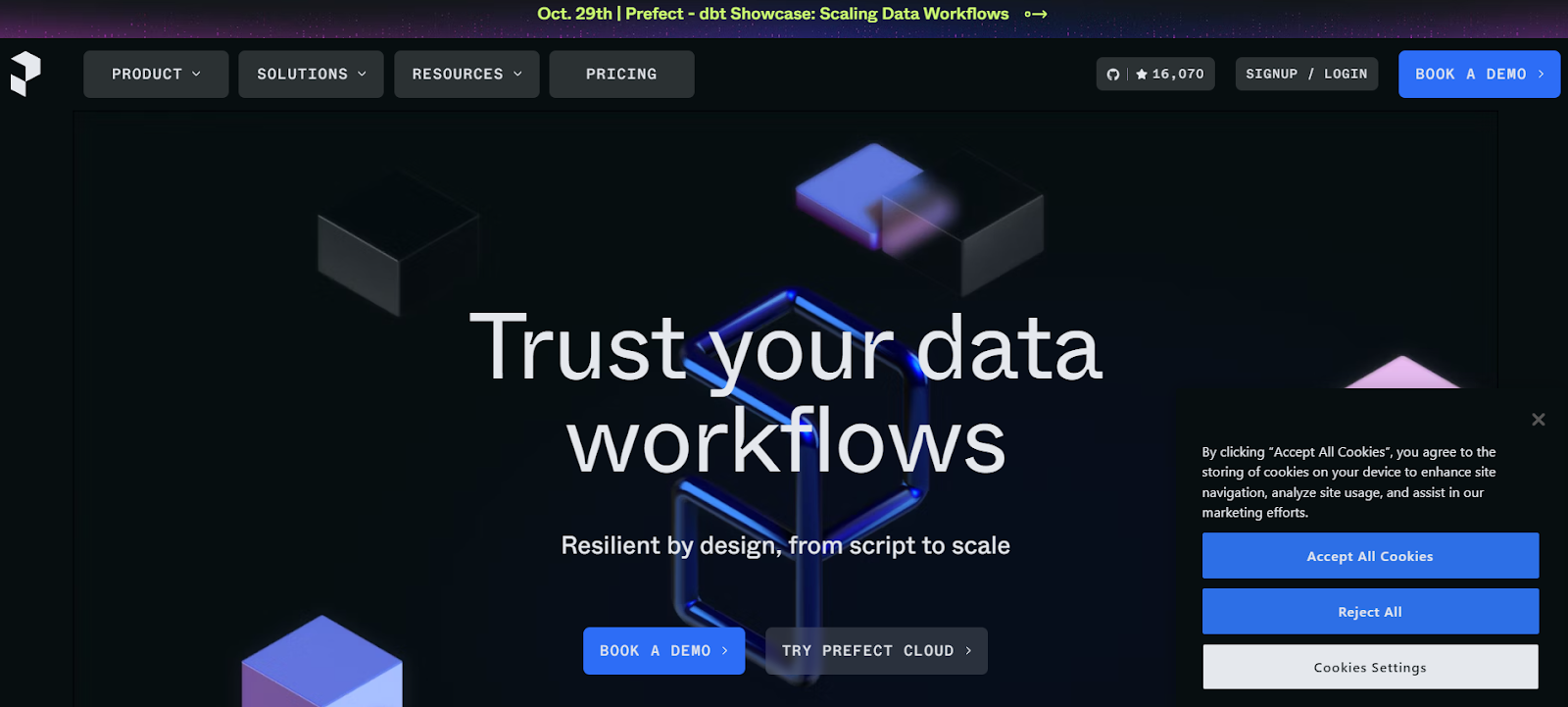
Image Source: Prefect
Prefect is an open-source framework that focuses on simplifying workflow automation through its intuitive UI built upon Python libraries such as Pandas and NumPy for fast processing speeds with large datasets. In addition to its powerful desktop version, which is accessible through browsers on any device without installing anything locally, Prefect also offers cloud options, enabling seamless integration with popular cloud platforms such as AWS, GCP, and Azure.
4. Dagster
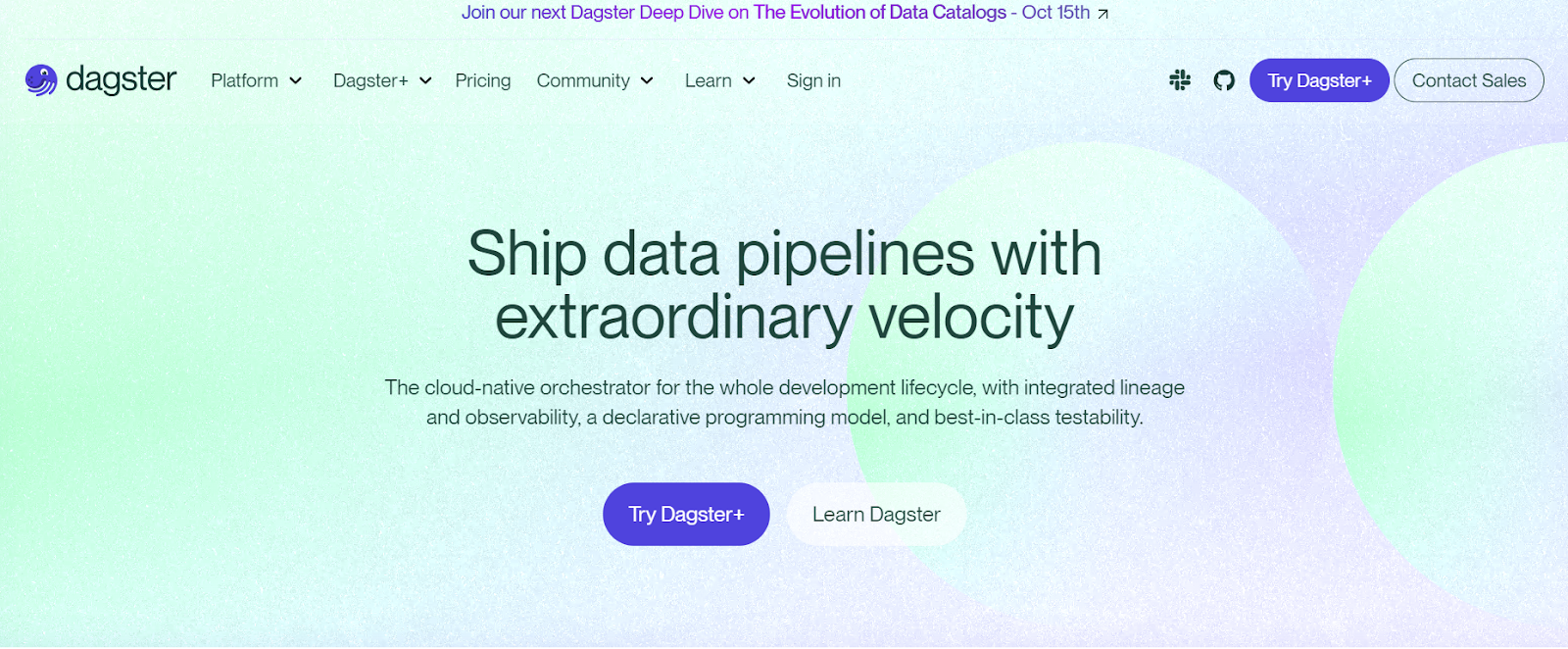
Image Source: Dagster
Dagster is an ML automation tool that supports data analytics workflows. It offers task scheduling, dependency management, data validation, and error handling features. Its user-friendly dashboard allows for easy monitoring of job status and logs, enabling quick troubleshooting in case of failure. With its large community of contributors, Dagster continuously improves its features and capabilities, making it a strong choice for those looking to manage their ML workloads.
5. Luigi

Image Source: Luigi
Developed by Spotify in 2012 as a proprietary system before being open-sourced in 2013, Luigi has been actively used in production environments by companies like Stripe and Asana. It utilizes a simple YAML configuration approach focused on flexibility rather than learning new scripting languages or using custom UIs on other tools. Notable features include a graphical display of dependencies between tasks and detailed logging mechanisms facilitating efficient debugging of critical issues.
These five data orchestration tools provide various options for managing and automating data workflows, whether for traditional analytics or machine learning applications. Ultimately, selecting which tool to use will depend on specific requirements and constraints. Considering their real-time capabilities, scalability, and ease of use, these tools are well worth exploring for any organization looking to optimize its data management processes..
Emerging Trends in Data Orchestration
With the advancement of technology and growing reliance on data-driven insights, there has been a significant shift in trends when it comes to data orchestration.
Real-Time Data Processing
One of the biggest emerging trends in data orchestration is real-time data processing. Traditional batch processing methods involve collecting and storing large amounts of data before analyzing it, resulting in delays and outdated insights. However, with the increasing availability of streaming technologies like Apache Kafka and Spark Streaming, businesses can process immense volumes of streaming data in real time. This enables organizations to take immediate actions based on up-to-date information instead of relying on historical data. Netflix utilized its subscriber data and real-time data analytics to transition into a global streaming business, as its revenues increased from $3.2 billion in 2011 to $33.7 billion by 2023.
Real-time processing also allows for continuous monitoring and tracking, which is particularly useful for industries such as finance or healthcare, where timely decisions can have significant impacts. With the rapid growth of the Internet of Things (IoT), real-time processing has become even more crucial as more devices create vast amounts of sensor-generated data that require immediate analysis.
Shift to Cloud-Based Technologies
Another trend in data orchestration is the move towards cloud-based technologies. As companies continue to gather massive amounts of complex and diverse datasets, traditional on-premise infrastructure needs help keeping up with storage demands or providing necessary scalability for dynamic workloads. Hence, more organizations are moving their data management operations onto cloud platforms such as Amazon Web Services (AWS), Savant, and Microsoft Azure.
A Deloitte survey found that cloud computing increased profits by 21% and growth by 26% for small and medium businesses. Cloud-based solutions offer an agile approach to managing big data by providing scalability options that cater to business requirements without exorbitant overhead costs associated with maintaining physical servers or storage facilities. These platforms also offer automated features like backup and recovery systems that ensure data integrity and minimize the risk of data loss.
Evolution from Monolithic to Microservices Architecture
The rise of microservices architecture has also affected data orchestration trends. Traditional monolithic software architectures involve a tightly coupled system with interconnected components, making it challenging to make changes or updates without impacting the entire system. Microservices, on the other hand, break down complicated applications into smaller, independent services that communicate with each other via APIs. The microservices architecture market is predicted to grow from $5.4 billion in 2022 to $21.61 billion by 2030.
Adopting a microservices approach for data orchestration results in a more flexible and modular system that allows for efficient handling of different data management tasks simultaneously. It also facilitates easier integration with new technologies as they emerge, allowing businesses to adapt to evolving market needs.
As technology advances rapidly, these trends will continue shaping data orchestration tools and providing businesses with better insights and decision-making capabilities.
Data Orchestration — A Foundation for Success
Data orchestration is crucial for organizations to efficiently manage large amounts of data and enable informed decisions based on factual and timely information. It provides a central platform for gathering, storing, and analyzing data from multiple sources, ensuring consistency and accuracy. Proper data orchestration helps organizations identify patterns and correlations between different datasets, uncovering hidden insights.
The need for advanced data orchestration tools is growing, offering opportunities for predictive analytics, security enhancements through blockchain technology, efficient big data handling, and automation through AI and machine learning. With the rise of IoT devices generating massive real-time data streams, real-time processing capabilities within data orchestration platforms are also needed.
Supercharge your data orchestration and analytics capabilities with Savant’s intuitive analytics automation platform. Say goodbye to siloed data and hello to seamless integrations with our data orchestration tools. Start optimizing your data orchestration today and watch your efficiency soar!
Also Read: Introduction to Using Automated Data Analytics in Business
FAQs
1. What is a data orchestration tool?
A data orchestration tool is software that automates collecting, organizing, and managing large volumes of data from various sources. It helps businesses streamline their data workflows, improving efficiency and decision making.
2. How do data orchestration tools differ from traditional ETL tools?
While ETL (Extract, Transform, Load) tools focus on moving data from one system to another, data orchestration tools have a broader scope. They extract and transform data and provide advanced scheduling, monitoring, and error-handling capabilities.
3. What are the advantages of using data orchestration tools?
Some essential advantages include increased productivity due to automated processes, reduced errors through standardization and consistency in data management, enhanced collaboration among teams working with different datasets, and improved data quality for more accurate insights.
4. Can businesses with small datasets benefit from using a data orchestration tool?
Yes! Data orchestration is not limited to large enterprises; even smaller businesses can benefit from these powerful tools. Data-driven decisions are becoming increasingly important across industries, so any organization dealing with multiple sources of information can benefit from these tools.
5. Do I require technical expertise to use a data orchestration tool?
While some technical knowledge may be required to set up and configure these tools initially, most platforms have user-friendly interfaces that make it easier for non-technical users to manage their workflows.
6. Are there any security problems when using third-party sources for my business's data?
This is a valid concern that businesses should address when choosing a data orchestration tool. Ensure your valuable data is protected with robust security measures like data encryption and compliance with industry standards such as GDPR and CCPA.
7. How does Savant differ from other data orchestration tools available in the market?
Savant goes beyond traditional ETL processes to offer complete end-to-end solutions for complex enterprise-level data management needs. Our platform provides advanced features such as automated workflows, self-service analytics capabilities, and integration with various cloud environments.
8. Can Savant cater to different industries' specific requirements?
Yes! We understand that every industry has unique data needs; our platform is designed to be adaptable to meet different business requirements.
9. How does Savant ensure the security of my organization's sensitive information?
At Savant, we prioritize data security by implementing industry-leading encryption methods and sticking to rigorous compliance standards such as HIPAA, SOC 2, and ISO 27001.